Service robots are developed to assist in both home and commercial settings. These robots collaborate with humans to accomplish a variety of tasks, and their design emphasizes safety and adaptability. To effectively perform their roles, they require capabilities such as voice and image recognition, manipulation skills, human-robot interaction, and flexible task-planning systems. Furthermore, foundational models for service robots have been developed to enable more advanced autonomous movement. The ability of the service robots is tested in competitions such as RoboCup (in a home setting) and World Robot Summit (WRS) (in a convenience store setting).
As artificial intelligence continues to progress, so too does the performance of robotic vision systems and task planning. Computational methods tailored for service robots are vital, particularly since these units typically operate on battery power. There have been significant advancements in both large AI models suited for cloud computing and lightweight AI models designed for edge computing, thereby enhancing their functionality and efficiency.
Incorporating the latest technologies can enable the completion of tasks within a controlled environment. However, the development of robots for competitions and the social implementation of service robots presents challenges, especially when it comes to adapting to unfamiliar settings. In this organized session, we intend to discuss robot hardware, system integration, AI models, and its implimentation methods for service robots.
Topics
- Hardware design for service robots
- System integration for service robots
- Machine learning for service robots
- Generative AI for service robots
- Foundation model for service robots
- Embedded system for service robots
- Human-robot interaction
- Competition outcome from RoboCup and WRS
Important Dates
Paper submission | 2025-04-10 |
Paper Submission
Please submit your paper by following the paper submission procedure of ICIEV.
Organizers
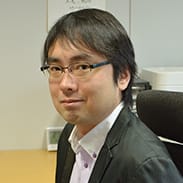
Hakaru Tamukoh
Kyushu Institute of Technology
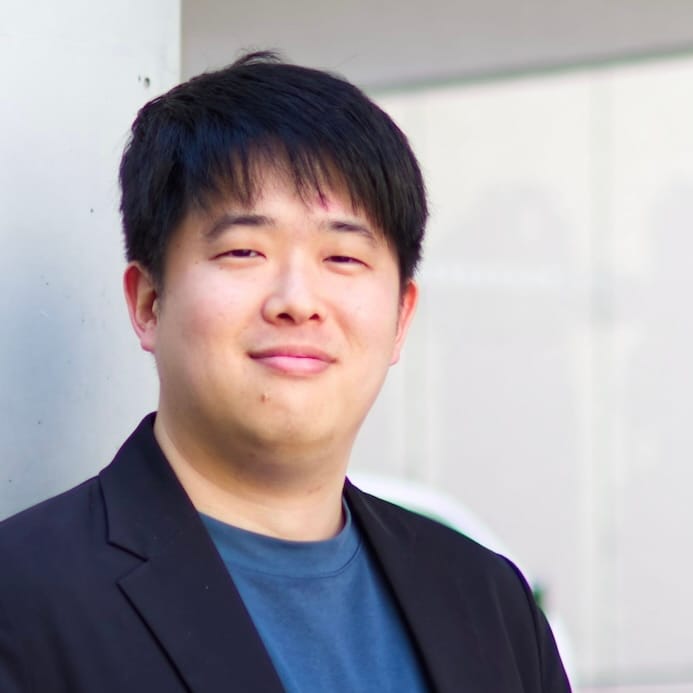
Yuichiro Tanaka
Kyushu Institute of Technology
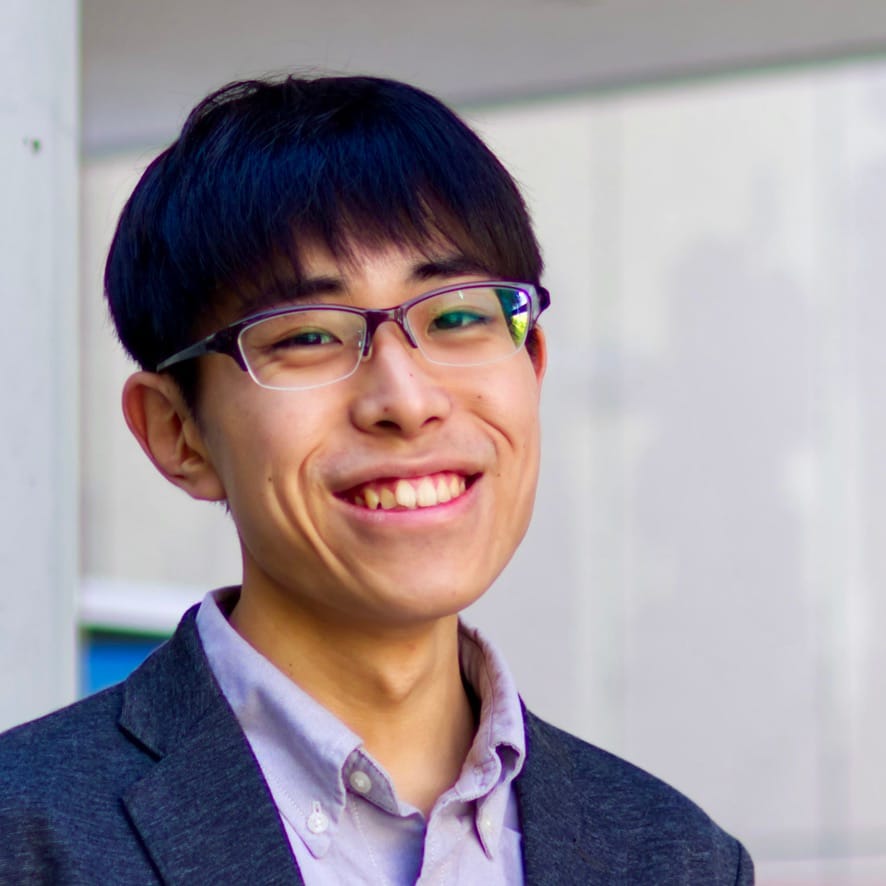
Akinobu Mizutani
Kyushu Institute of Technology